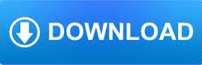
Application of the APSIM model to exploit G × E × M interactions for maize improvement in Ethiopia. Replication of this study is necessary to further define the limitations and opportunities of the developed system. Seyoum S, Rachaputi R, Chauhan Y, Prasanna B, Fekybelu S. Extending the scope of previous work, our results demonstrate the power of data assimilation to constrain important model estimates beyond the assimilated state variable, such as nitrate leaching. Forecasts of aboveground measures did not dramatically change with assimilation, a fact which highlights the limited potential of soil moisture as a constraint for a site with no water stress. Such improvements led to changes in the model's soil water and nitrogen processes and, on average, increased accuracy in forecasts of annual tile flow by 43% and annual nitrate loads by 10%.
APSIM R FREE
The system improved the accuracy and precision of soil moisture estimates for the assimilation layers by an average of 42% and 48%, respectively, when compared to the free model. We tested this system at the Energy Farm, a well-monitored research site in central Illinois, where we assimilated observed in situ soil moisture at daily time steps for two years and evaluated how assimilation impacted model forecasts of soil moisture, yield, leaf area index, tile flow, and nitrate leaching by comparing estimates with in situ observations.
APSIM R UPDATE
Our final novel system utilizes the Ensemble Kalman Filter to constrain model states and update model parameters at observed time steps and incorporates an algorithm that improves system performance through the joint estimation of system error matrices. To overcome the limitations of process-based models and observed data, we iteratively designed and tested a generalizable and robust data-assimilation system that systematically constrains state variables in the APSIM model to improve forecast accuracy and precision. Yet, the accuracy and precision of our forecasts remains limited by current tools and methods. Write.table(dat, "met.met", append=T, sep=paste(rep(" ",5), collapse=""), row.names=F, quote=F) #appending to that, work with how many spaces you need, I used 5 hereĪdding "row.As we face today's large-scale agricultural issues, the need for robust methods of agricultural forecasting has never been clearer. Tav = ", tavC," (oC) ! annual average ambient temperatureĪmp = ",ampC," (oC) ! annual amplitude in mean monthly temperature") I guess this answers your question, you can use all your header here, no need to open separately and work on that. Once I have all of this data made up in dataframes, all I want to do is write them into a format my program will recognize (.met). Here is what a few lines of your average met file looks like: year day radn maxt mint rain Official Documentation and File Format Description
APSIM R DOWNLOAD
Here you can download presentations used on the course. The main focus of the lectures were APSIM application in nitrogen and forage crop modeling. Lectures soon turned into a workshop, since a lot of people from PFNS were interested. Seed yield across all sites was predicted by the APSIM model. Manschadi from BOKU gave a course for PhD students for APSIM use. txt and want to end up with a file that is. Predicted days to flowering (APSIM stage 6.0-start of flowering) (R 2 0.9) were correlated with field observations from the multi-environment trials, despite field observation being qualitatively rated (). I am trying to convert some directions for Excel to an R script. I am looking for some one who is knowledgeable about both R and file formats/extensions. This is the first published example of extending APSIM P routines to another crop (beans) from maize.
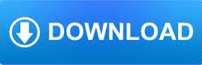